Agentic AI Explained: The Impact of AI's Next Giant Leap
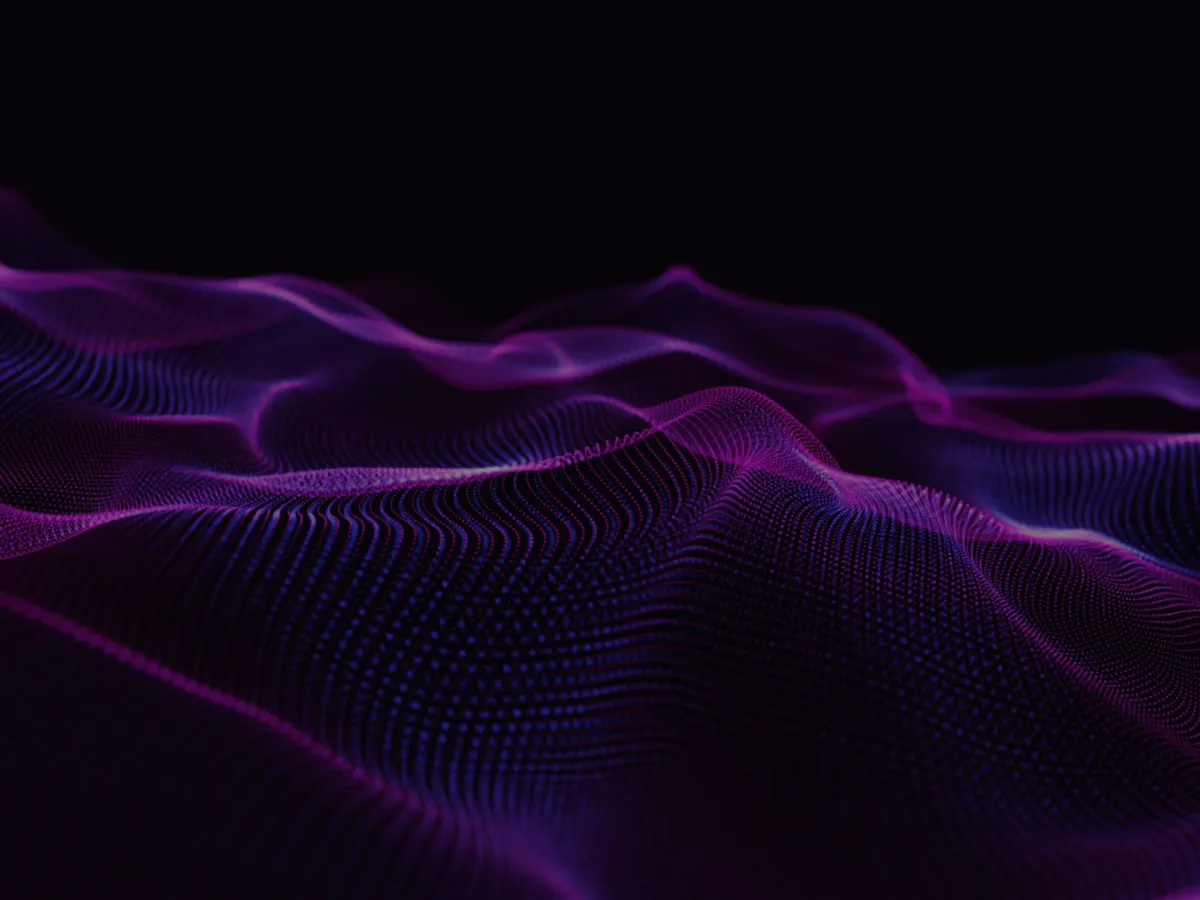
Introduction
Artificial intelligence is evolving at an unprecedented pace, and one of its most transformative advancements is agentic AI. Unlike traditional AI models that follow predefined rules or require continuous human oversight, agentic AI has the capability to autonomously pursue complex goals, make decisions, and learn from interactions—all with minimal human intervention.
This leap in AI innovation is reshaping industries, optimizing workflows, and introducing new possibilities for automation. But what is agentic AI, and how does it differ from the AI systems we are familiar with today? Let’s explore its characteristics, applications, and the challenges that come with its implementation.
What is Agentic AI?
Agentic AI refers to AI systems that can operate independently, execute multi-step tasks, and adapt dynamically to changing environments. Unlike conventional AI, which follows strict algorithms and predefined rules, agentic AI continuously learns, reasons, and makes decisions based on context and feedback.
Key Characteristics of Agentic AI
- Autonomy: Capable of initiating and completing tasks with minimal human input.
- Reasoning: Makes sophisticated decisions by analyzing trade-offs and evaluating outcomes.
- Reinforcement Learning: Improves over time by interacting with its environment and refining its strategies.
- Language Understanding: Comprehends and follows complex instructions in a contextual manner.
- Workflow Optimization: Efficiently manages multi-step processes across different domains.
Agentic AI combines the flexibility of large language models (LLMs) with the precision of traditional programming. It leverages machine learning (ML), natural language processing (NLP), and real-time data processing to handle intricate workflows that demand autonomous decision-making.
Agentic AI vs. Generative AI: Key Differences
Agentic AI and Generative AI are two distinct branches of artificial intelligence, each with unique functionalities and applications.
Feature | Agentic AI | Generative AI |
---|---|---|
Primary Function | Executes tasks, makes decisions, and operates autonomously | Generates new content (text, images, audio, etc.) based on patterns |
Learning Approach | Learns from real-world interactions, adapts strategies over time | Trained on historical data, generates outputs but doesn’t adapt dynamically |
Proactivity | Initiates actions, anticipates needs, and solves problems independently | Reacts to user prompts but does not take independent action |
Examples | AI-driven personal assistants, autonomous research agents, self-optimizing workflows | ChatGPT, DALL·E, MidJourney, AI-generated music or writing |
Use Case | Automating complex decision-making and workflows | Content creation, language translation, and image generation |
While Agentic AI focuses on autonomous problem-solving and decision-making, Generative AI specializes in producing creative outputs based on input data. In the future, these two AI types may complement each other—Agentic AI using Generative AI to create contextually relevant content, and Generative AI leveraging Agentic AI for more refined and goal-driven outputs.
Can Agentic AI Learn and Adapt Without Human Intervention?
Yes, agentic AI is designed to continuously learn, evolve, and adapt—a fundamental trait that sets it apart from conventional AI.
How Agentic AI Achieves This:
- Cognitive Autonomy: Initiates and completes operations independently, making real-time decisions.
- Reinforcement Learning: Learns from experiences rather than relying solely on deterministic programming.
- Perceive-Reason-Act-Learn Cycle: Gathers data, makes decisions, takes action, and evaluates outcomes for continuous improvement.
- Proactive Interaction: Engages with external environments to adjust in real-time.
- Self-Optimization: Adjusts strategies and refines decision-making without requiring manual updates.
Real-World Applications of Agentic AI
Agentic AI is already transforming industries by enhancing efficiency, decision-making, and automation. Some notable agentic AI examples include:
- Healthcare: AI-driven diagnosis, treatment planning, and real-time patient monitoring (e.g., Google’s AI for Breast Cancer Diagnosis).
- Smart Assistants: Voice-controlled AI assistants like Amazon Alexa and Google Assistant that learn user preferences.
- Fraud Detection & Cybersecurity: Systems like Darktrace that identify threats and autonomously block suspicious activity.
- Marketing & Advertising: AI-powered personalized ad targeting used by Netflix, Spotify, and Salesforce.
- Supply Chain Optimization: AI logistics management by companies like FedEx.
- Autonomous Vehicles: Self-driving cars from Waymo making real-time navigation decisions.
- Robotic Process Automation (RPA): Automating repetitive business tasks to increase efficiency.
- Smart Manufacturing: AI-driven predictive maintenance and process optimization (Siemens, AVEVA).
- Energy Management: Smart grid optimization using AI (Google DeepMind, Duke Energy).
What is an Agentic AI Framework?
An agentic framework provides the foundation for developing AI agents. These frameworks offer:
- Pre-Built Components: Ready-made modules for seamless AI development.
- Communication Protocols: Enables AI agents to interact efficiently.
- Planning & Reasoning: Helps AI agents analyze data, set goals, and execute strategies.
- Monitoring & Debugging Tools: Tracks AI performance and ensures reliability.
Top Agentic AI Frameworks:
Framework | Key Features | Use Cases |
---|---|---|
Microsoft AutoGen | Multi-agent orchestration, real-time task delegation | Data-driven applications, automation |
LangChain | LLM-driven workflows, memory management | Dynamic applications, adaptable tasks |
Hugging Face Transformers 2.0 | High-performance workflows, secure execution | Cross-domain AI applications |
MetaGPT | Multi-agent collaboration with role-based tasking | Software development, competitive analysis |
CrewAI | Role-based multi-agent coordination | Research, large-scale data processing |
These agentic AI frameworks enable developers to build intelligent AI agents capable of autonomous reasoning and collaboration.
Challenges of Implementing Agentic AI
While agentic AI holds immense potential, its implementation comes with challenges, including:
- Technical Complexity – Requires advanced architectures and significant computational power.
- Data Privacy & Security – Processing vast amounts of sensitive data raises privacy concerns.
- Bias & Fairness – AI may inherit biases from training data, leading to unfair outcomes.
- Transparency & Explainability – Complex decision-making can create "black box" AI concerns.
- Unpredictability – AI can evolve in unintended ways, making error diagnosis difficult.
- Ethical Considerations – Questions around accountability and moral decision-making arise.
- Malicious Use Risks – AI could be exploited for cyberattacks or misinformation.
Conclusion
Agentic AI represents a significant leap forward in AI capabilities, offering autonomous decision-making, adaptability, and real-time problem-solving. From healthcare and cybersecurity to marketing and autonomous systems, its applications are vast and growing. However, with great power comes great responsibility—ensuring ethical deployment, minimizing biases, and maintaining transparency will be critical in shaping a future where agentic AI works in harmony with human intelligence.